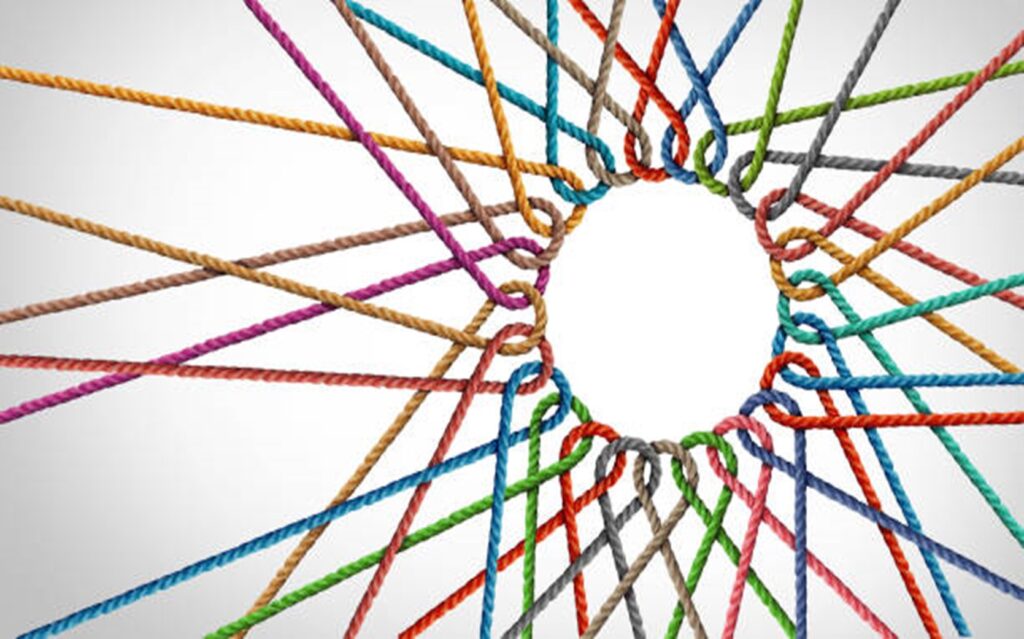
Responsible Artificial intelligence (AI)
AI is revolutionizing industries, but with great power comes great responsibility. For organization implementing AI, ensuring control and ethical implementation is crucial. We become what we think, we become what we are based on our innate nature , our environment, our morals and values and also our intellect, mind and energy. Same is true with any development, till now we have grown our organization, build their culture and principle , focused on providing them environment for sustainable growth . Now is the time to scale this to our ecosystem at large, responsibility is very big for those organization who have knowhow and capital and vision to do so and are building AI to solve simple to complex problem which has far reaching impact. AI should be for good and growth only. Here are some control considerations to build AI responsibly.
Ethics and governance:
Define AI’s Purpose: Organization should define a clear purpose for AI that aligns with overall good. This purpose should guide every stage of development, ensuring the AI serves humanity and avoids causing harm. The board should develop a mission statement for your AI that emphasizes its positive societal impact.
Focus on Impact, Not Just Profit :AI development shouldn’t be driven solely by profit or personal gain. Instead, organization should focus on creating AI solutions that benefit society as a whole. They should evaluate potential develop AI projects based on their contribution beyond financial potential for sustainable growth.
Build Fair and Objective : They should develop AI that is free from biases and emotional influences. This ensures fair and objective decision-making. they should use diverse personnel, datasets and fairness metrics to train AI, mitigating potential biases in its decision-making process.
Embrace Safe and Ethical Practices: Make commitment to responsible innovation, acknowledging the potential impact of AI and building safeguards. To achieve same conduct regular risk assessments and implement controls (like human oversight) to prevent misuse or unintended consequences of your AI.
Establish an AI Governance Board: This boards should be composed of diverse stakeholders, should oversee AI development and deployment, ensuring alignment with ethical principles. AI should be fair, accountable, and transparent, minimizing harm and bias while respecting human values fundamental rights and privacy.
Develop a Code of Ethics for AI: This code should outline commitment to fairness, transparency, accountability, and privacy. It should guide to strive for design and deploying AI systems that minimize bias and promote equal treatment for all. Build AI systems that is transparent in their decision-making processes, allowing for human oversight and understanding. There should be accountability or the development, deployment, and use of AI, with clear lines of responsibility. Company should prioritize user privacy, safeguarding personal data and adhering to all relevant regulations.
Clear Value Training and Measurement
Train on High-Quality, Diverse Data: Biased data leads to biased AI. Organization should focus on gathering data that represents target audience or scenario to avoid discrimination.
Measure for Fairness and Explainability: Use fairness metrics to detect bias in your AI’s outputs. Implement Explainable AI (XAI) techniques to understand how your AI arrives at decisions .
Prioritizing User Autonomy: Transparency in Recommendation Systems: AI system should allow user to understand the factors influencing suggestions and providing options to refine or opt-out altogether.
Controlling Bias and Ensuring Transparency
Human-in-the-Loop Systems: Organization should Incorporate human oversight for critical decisions, allowing for intervention if bias is detected. For same it should regularly analyze model outputs for statistical bias using fairness metrics (e.g., parity, equality of opportunity). Set thresholds for bias metrics to trigger alerts when potential bias is detected. Integrate human review processes for flagged outputs, allowing for correction and bias mitigation strategies. Regularly audit your AI systems for bias and fairness. Encourage user feedback to identify potential issues and Continuously refine model training data and algorithms based on identified bias and human feedback
Technical Controls: Organization may Integrate Explainable AI (XAI) libraries like LIME(Local Interpretable Model-Agnostic Explanations) or SHAP (SHapley Additive exPlanations) to explain AI model’s predictions in a human-understandable way. This can help identify potential biases in the model’s decision-making process.
Data Validation and Verification: AI implementation should incorporate data validation techniques to ensure data accuracy and consistency. Use data fingerprinting to verify the source of data and prevent manipulation
Bias Detection and Mitigation
Debiasing Techniques : Entity can explore to use techniques like adversarial debiasing to identify and remove bias from training data.
Fairness-Aware Metrics: They can incorporate fairness metrics like statistical parity or equal opportunity into AI model training process to actively mitigate bias .
Blind Reviews: They could conduct blind reviews of AI model’s development process to identify potential biases introduced by designers, developers, or governance personnel.
Security and Privacy Controls
Differential Privacy: AI implementation may inject carefully calculated noise to data during training using differential privacy techniques. This protects individual privacy while preserving the data’s utility for training the AI model. This provide greater mathematical accuracy to privacy protection.
Vulnerability Assessments and penetration testing : Entity should regularly conduct vulnerability assessments on AI systems to identify and patch potential security weaknesses that could be exploited for malicious purposes. It should Simulate cyberattacks through penetration testing to assess the robustness of your AI systems against unauthorized access or manipulation.
Preventing Misuse and Malfunction
Risk Assessments: Those responsible for AI should Conduct comprehensive risk assessments to identify potential risks associated with AI, such as bias, security, safety, explainability, transparency, and societal impact . This will enable to manage risk of AI and ensure responsible development and deployment.
Safety by Design: it should embed safety considerations into the entire AI development lifecycle, from design to deployment. It should implement to address risk throughout development (data, models, testing), deployment (monitoring, human oversight), and adapting as needed.
Adversarial Robustness Training: It should train AI model to be robust against adversarial attacks such as evasion, poisoning or model extraction attack, which can manipulate inputs to cause the AI to malfunction.
Conclusion :
To put it together some control consideration
Align AI with values: Define a clear purpose focused on positive societal impact.
Respect for Fundamental Rights: Ensure your AI adheres to EU regulations regarding fundamental rights, such as privacy, non-discrimination, and fairness.
Diverse Team: Assemble a team with varied backgrounds and perspectives to mitigate bias.
Develop a Responsible AI Strategy: Outline entities commitment to responsible AI development and deployment.
Train Your Team: Educate team on ethical AI principles, bias detection techniques, and security best practices.
Clean Data Foundation: Collect high-quality, unbiased data reflecting your target audience.
Use Pre-Vetted Datasets: Consider using pre-vetted datasets from reputable sources to reduce bias risk
Transparency is Key: Use XAI to understand decision-making and educate users about how your AI works
Implement Explainable AI Tools: Explore open-source XAI libraries to make your AI’s decision-making process more transparent.
Safety ,Security and privacy by design : Perform risk assessment and ensure security , safety and privacy. Refer framework like NIST AI framework and ISO 42001
Human in Loop and regular review: Build Human in loop for intervention and regularly monitor.
Start Small, Iterate Often: Launch responsible pilot projects, gather feedback, and continuously refine your model
Partner with AI Ethics and risk management Experts: Seek guidance from AI ethics and risk management experts to ensure you’re on the right track.
By implementing these control measures, organization can ensure their AI is developed to be used responsibly, ethically, and transparently. We need to Remember, responsible AI is not just good for society, it’s also good for business, fostering trust and building a sustainable future for your AI-powered venture.
Reference
- OECD AI Principles: https://oecd.ai/en/ai-principles
- NIST AI Framework: https://www.nist.gov/artificial-intelligence
- ISO 42001: https://www.iso.org/standard/81230.html